Published: Cross-Architecture Tuning of Si Qubit Devices using Machine Learning
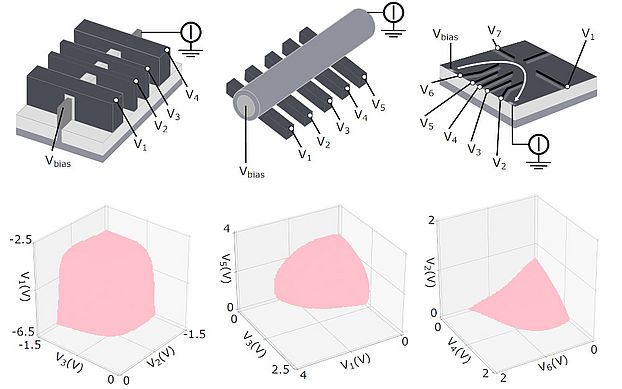
Device-to-device variability and disorder in spin qubits makes it currently necessary to individually tune each device in a large-dimensional gate space to find the optimal conditions for qubit operation. We take a key step with a machine-learning algorithm that is capable of tuning a 4-gate Si FinFET, a 5-gate GeSi nanowire and a 7-gate SiGe heterostructure device from scratch, also providing insight into the parameter space landscapes for each of these materials (see Figure above). These results show that overarching solutions for the tuning of quantum devices are are possible with machine learning.
Collaboration of Oxford University, IST Austria, and the University of Basel.
Supported in Basel by the NCCR SPIN of the Swiss NSF, the EU H2020 European Microkelvin Platform (EMP), and the Swiss Nanoscience Institute (SNI).
Cross-architecture Tuning of Silicon and SiGe-based Quantum Devices Using Machine Learning
B. Severin, D. T. Lennon, L. C. Camenzind, F. Vigneau, F. Fedele, D. Jirovec, A. Ballabio, D. Chrastina, G. Isella, M. de Kruijf, M. J. Carballido, S. Svab, A. V. Kuhlmann, F. R. Braakman, S. Geyer, F. N. M. Froning, H. Moon, M. A. Osborne, D. Sejdinovic, G. Katsaros, D. M. Zumbühl, G. A. D. Briggs, and N. Ares, Scientific Reports 14, 17281 (Jul 27, 2024, open access).
manuscript pdf, supplementary pdf. data not open (older paper)