Published in PRX: Bridging the Reality Gap in Quantum Devices with Machine Learning
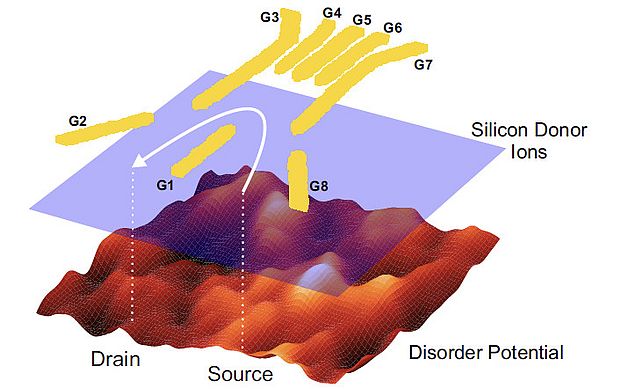
The discrepancies between reality and simulation impede the optimization and scalability of solid-state quantum devices. Disorder induced by the unpredictable distribution of material defects is one of the major contributions to the reality gap.
In this study, we bridge this gap using physics-aware machine learning, in particular, using an approach combining a physical model, deep learning, Gaussian random field, and Bayesian inference. This approach enables us to infer the disorder potential of a nanoscale electronic device from electron-transport data. This inference is validated by verifying the algorithm’s predictions about the gatevoltage values required for a laterally defined quantum-dot device in AlGaAs/GaAs to produce current features corresponding to a double-quantum-dot regime.
The inference algorithm can be easily scaled to larger, more complex devices. In this way, our approach could be used to study the variability of devices in large arrays, extending over micro- and millimeter areas.
Experiments by the Ares group (Oxford University) on Basel GaAs quantum dots (Zumbühl group), also supported by the EU FP7 RIA European Microkelvin Collaboration and the NCCR SPIN of the Swiss NSF.
Bridging the Reality Gap in Quantum Devices with Physics-Aware Machine Learning
D. L. Craig, H. Moon, F. Fedele, D. T. Lennon, B. Van Straaten, F. Vigneau, L. C. Camenzind, D. M. Zumbühl, G. A. D. Briggs, M. A. Osborne, D. Sejdinovic, N. Ares,
Phys. Rev. X14, 011001 (Jan 4, 2024), supplementary pdf